





Fully automated data curation
We provide a fully automated data curation service which can be used to enhance the quality of raw clinical, genetic and lifestyle data. The service supports functionalities for metadata extraction, outlier detection, data imputation, and de-duplication.
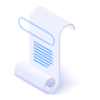
Flexible performance and scale
Both conventional methods, such as, the z-score and the interquartile range, as well as, advanced methods, such as, the isolation forests and the Gaussian elliptic curves are used to detect anomalies within the raw data.
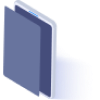
Advanced data imputers
Advanced data imputers are used to replace the missing values based on virtual patient profiles which are built for each real patient. Similarity detection methods and lexical matchers are used to identify variables with identical distributions and common meaning. Useful metadata are provided to the user along with detailed reports where the inconsistent fields are highlighted with color coding
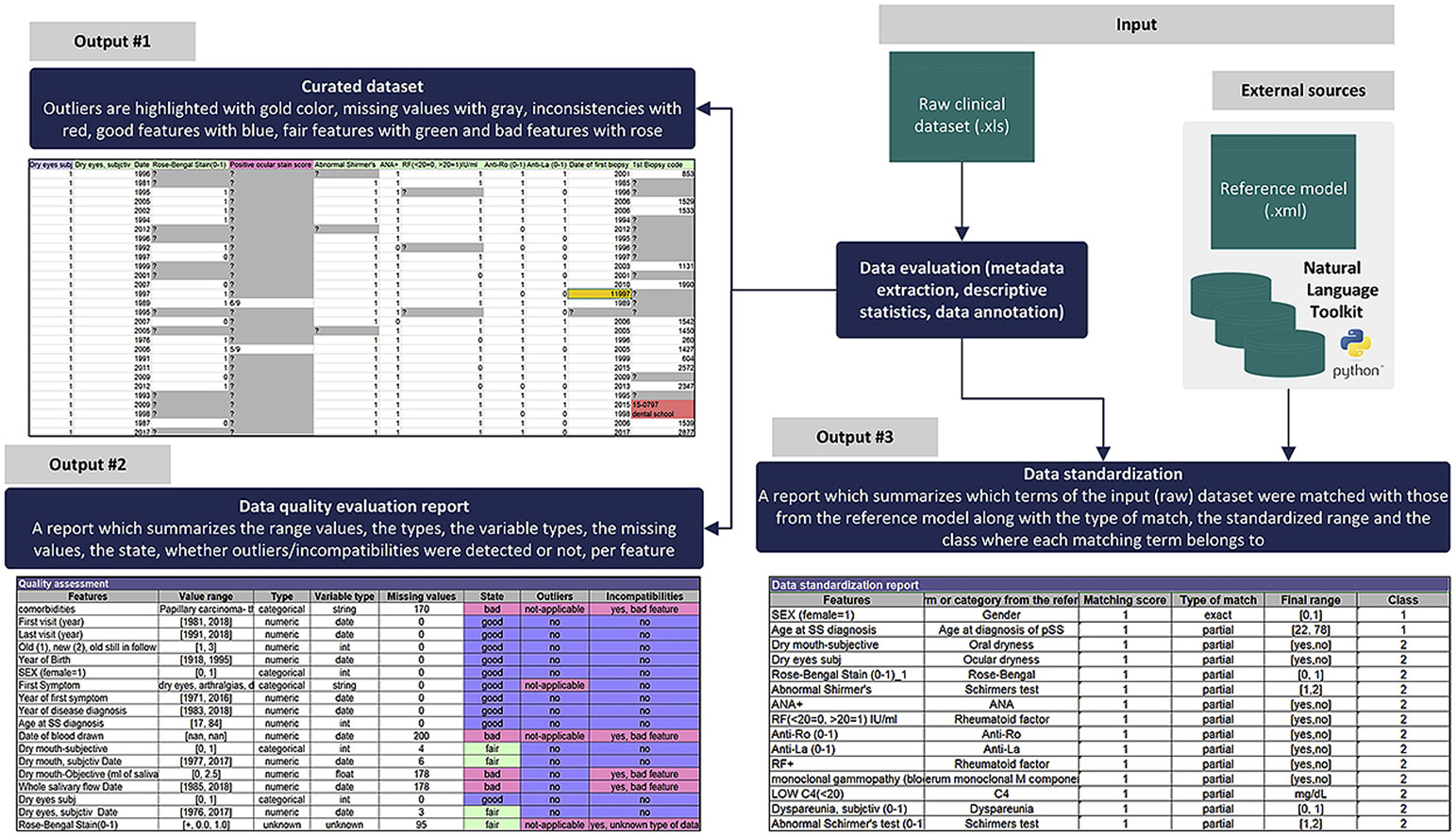
Pezoulas VC, Kourou KD, Kalatzis F, Exarchos TP, Venetsanopoulou A, Zampeli E, Gandolfo S, Skopouli F, De Vita S, Tzioufas AG, Fotiadis DI. Medical data quality assessment: On the development of an automated framework for medical data curation. Comput Biol Med. 2019 Apr;107:270-283. doi: 10.1016/j.compbiomed.2019.03.001. Epub 2019 Mar 7. PMID: 30878889.
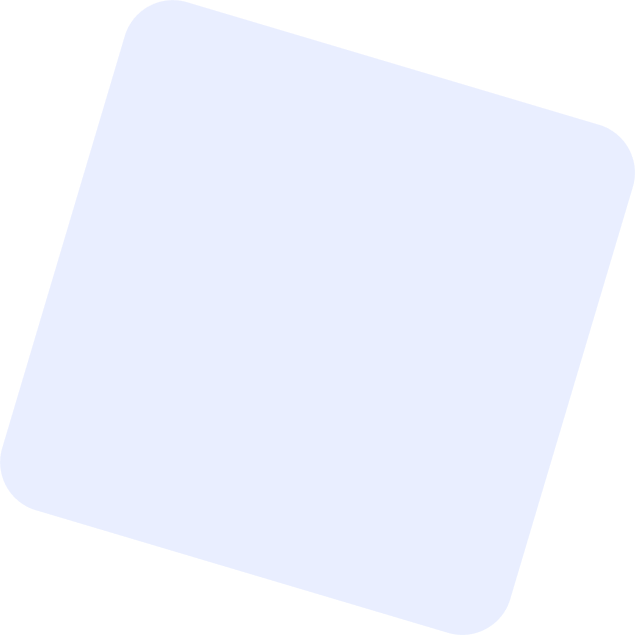
Data quality assessment has gained attention in the recent years since more and more companies and medical centers are highlighting the importance of an automated framework to effectively manage the quality of their big data. Data cleaning, also known as data curation, lies in the heart of the data quality assessment and is a key aspect prior to the development of any data analytics services. In this work, we present the objectives, functionalities and methodological advances of an automated framework for data curation from a medical perspective. The steps towards the development of a system for data quality assessment are first described along with multidisciplinary data quality measures. A three-layer architecture which realizes these steps is then presented. Emphasis is given on the detection and tracking of inconsistencies, missing values, outliers, and similarities, as well as, on data standardization to finally enable data harmonization. A case study is conducted in order to demonstrate the applicability and reliability of the proposed framework on two well-established cohorts with clinical data related to the primary Sjögren’s Syndrome (pSS). Our results confirm the validity of the proposed framework towards the automated and fast identification of outliers, inconsistencies, and highly-correlated and duplicated terms, as well as, the successful matching of more than 85% of the pSS-related medical terms in both cohorts, yielding more accurate, relevant, and consistent clinical data.