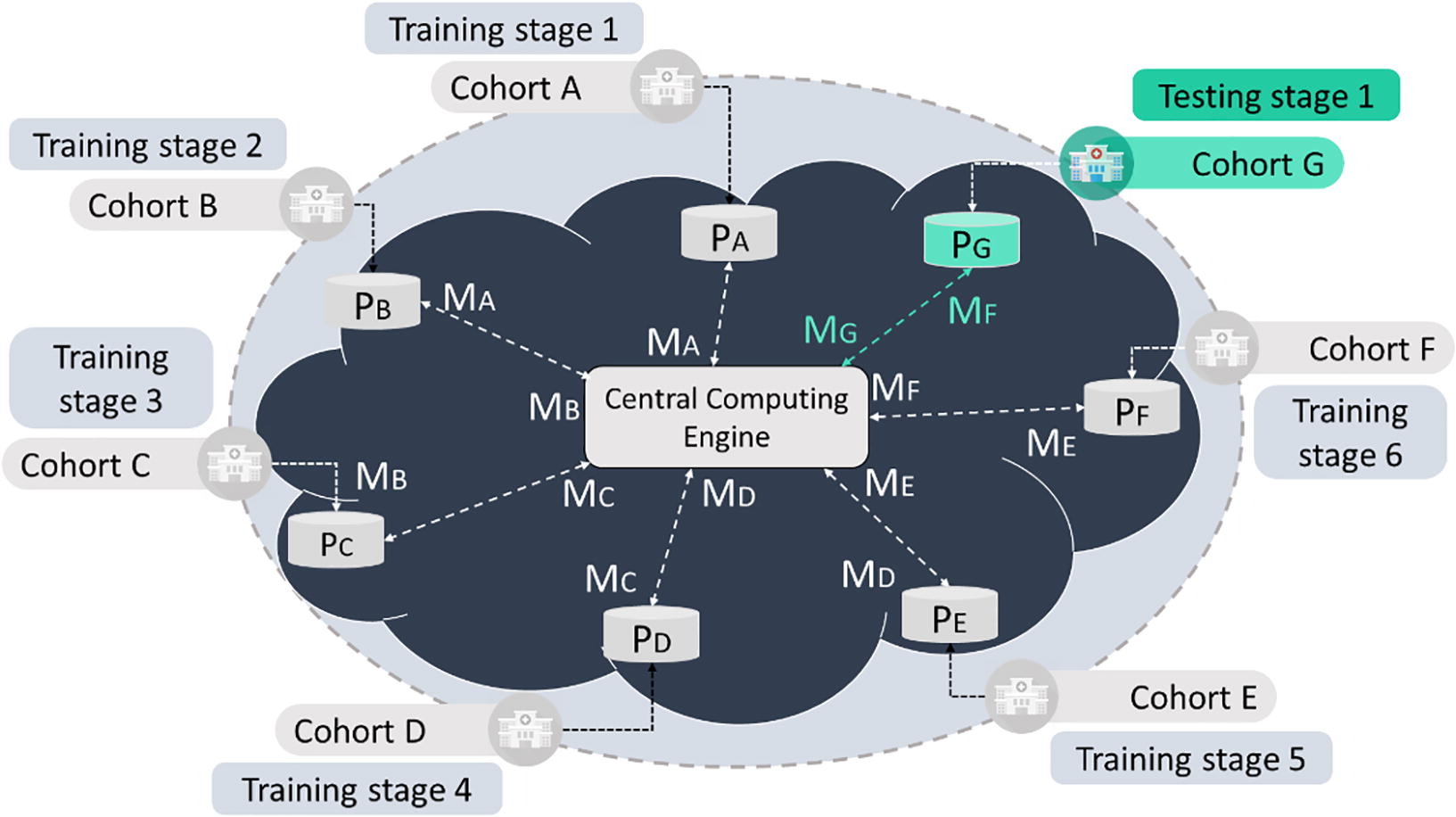
An illustration of the federated AI model training and testing workflow, where the testing cohort is depicted in green color.
Pezoulas VC, Goules A, Kalatzis F, Chatzis L, Kourou KD, Venetsanopoulou A, Exarchos TP, Gandolfo S, Votis K, Zampeli E, Burmeister J, May T, Marcelino Pérez M, Lishchuk I, Chondrogiannis T, Andronikou V, Varvarigou T, Filipovic N, Tsiknakis M, Baldini C, Bombardieri M, Bootsma H, Bowman SJ, Soyfoo MS, Parisis D, Delporte C, Devauchelle-Pensec V, Pers JO, Dörner T, Bartoloni E, Gerli R, Giacomelli R, Jonsson R, Ng WF, Priori R, Ramos-Casals M, Sivils K, Skopouli F, Torsten W, A G van Roon J, Xavier M, De Vita S, Tzioufas AG, Fotiadis DI. Addressing the clinical unmet needs in primary Sjögren’s Syndrome through the sharing, harmonization and federated analysis of 21 European cohorts. Comput Struct Biotechnol J. 2022 Jan 7;20:471-484. doi: 10.1016/j.csbj.2022.01.002. PMID: 35070169; PMCID: PMC8760551.
Federated Learning
PRECIOUS advanced federated AI model deployment framework: PRECIOUS provides a unique federated AI model deployment framework which uses incremental learning to build trustworthy AI models across data that are stored in private databases within a cloud environment. To do so, we use a central node to orchestrate the training of advanced federated AI algorithms across multiple nodes (i.e., cloud databases). The patient data are stored in secure private cloud databases. The central node utilizes the federated AI model deployment phase, where a federated learning algorithm is first trained on a private cloud database, say A and then the model weights are incrementally updated across the rest of the private databases. Class imbalance handling functionalities and explainability methods are also provided as part of the overall federated AI model deployment framework.